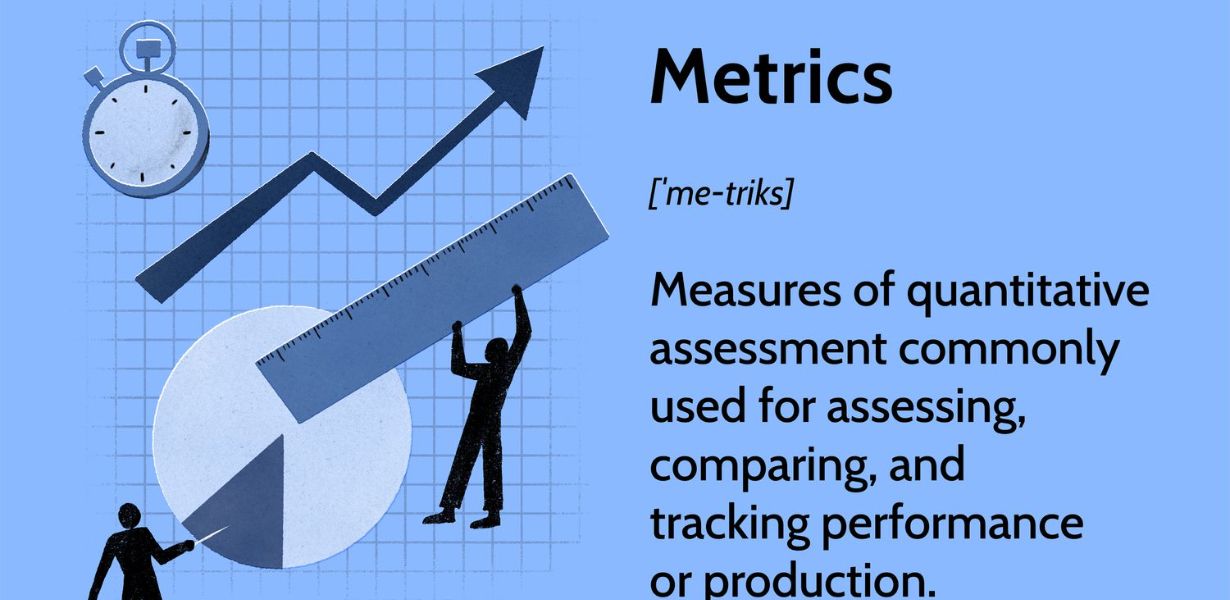
Decoding AI Performance Metrics for Strategic Insights
- Post
- August 9, 2023
- Ad Serving Tech, Dynamic Creative Optimization, Metrics
- 0 Comments
As the technological landscape continues to evolve, artificial intelligence (AI) stands at the forefront of innovation. From enhancing customer experiences to optimizing business processes, AI’s impact is undeniable. However, to harness its potential, we must delve into the realm of AI performance metrics. These metrics provide us with valuable insights into the effectiveness and efficiency of AI systems, helping us make informed decisions. In this article, we’ll embark on a journey to uncover the key metrics that crack the code of AI performance.
Defining AI Performance Metrics
AI performance metrics are quantifiable measurements used to evaluate the success of AI models, applications, and systems. These metrics go beyond traditional assessments and offer a comprehensive understanding of AI’s capabilities. Let’s explore some of the most critical metrics:
Accuracy and Precision
In the intricate world of AI, accuracy and precision are fundamental. Accuracy gauges the proportion of correctly predicted outcomes, while precision measures the model’s ability to correctly predict positive cases. Balancing these metrics is essential for reliable AI systems.
Key Points:
Striking the right balance between accuracy and precision prevents false positives and false negatives.
AI models should be fine-tuned to achieve optimal accuracy and precision based on the application’s requirements.
Recall and F1 Score: Beyond the Basics
Recall
Recall, also known as sensitivity or true positive rate, assesses the model’s ability to identify all relevant instances within a dataset. It’s particularly crucial when dealing with scenarios where missing potential positives could have severe consequences.
Key Points:
High recall is essential in healthcare diagnostics and fraud detection to minimize missed opportunities.
Recall should be considered alongside precision to strike the right balance between sensitivity and accuracy.
F1 Score
The F1 score harmonizes precision and recall, offering a comprehensive evaluation of an AI system’s performance. It considers both false positives and false negatives, making it a valuable metric for tasks with imbalanced class distributions.
Key Points:
F1 score is suitable for scenarios where achieving high precision and recall simultaneously is crucial.
It’s especially relevant in sentiment analysis and anomaly detection.
Area Under the ROC Curve (AUC-ROC)
AUC-ROC
The AUC-ROC metric evaluates a model’s ability to distinguish between positive and negative instances across various threshold settings. It provides insights into the model’s discriminative power and is widely used in binary classification tasks.
Key Points:
AUC-ROC values closer to 1 indicate superior model performance in distinguishing between classes.
AUC-ROC is useful in medical diagnosis and credit risk assessment.
Mean Absolute Error (MAE) and Mean Squared Error (MSE)
MAE and MSE
For regression tasks, MAE and MSE are go-to metrics. MAE calculates the average absolute difference between predicted and actual values, while MSE considers squared differences. These metrics quantify the model’s predictive accuracy.
Key Points:
MAE is less sensitive to outliers, making it suitable for datasets with extreme values.
MSE penalizes larger errors more significantly, making it suitable for applications where precision matters.
Computing Efficiency: Speed and Resource Usage
Inference Speed
AI systems’ efficiency goes beyond accuracy; speed matters too. Inference speed measures how quickly a model makes predictions, which is crucial for real-time applications like chatbots and autonomous vehicles.
Key Points:
Balancing accuracy and inference speed is a challenge, often requiring trade-offs.
Quantifying latency in milliseconds is essential for applications requiring quick responses.
Resource Usage
AI models’ resource consumption directly impacts their feasibility and scalability. Monitoring metrics like memory consumption and CPU utilization helps optimize resource allocation.
Key Points:
Efficient models ensure cost-effective and environmentally friendly AI implementations.
Resource usage optimization is crucial for edge computing and IoT applications.
Final Words
In the ever-evolving world of AI, deciphering performance metrics is essential for harnessing its full potential. By grasping the significance of accuracy, precision, recall, F1 score, AUC-ROC, and efficiency metrics, organizations can fine-tune their AI systems for optimal results. Balancing these metrics in alignment with specific applications ensures AI’s seamless integration into various domains.
Commonly Asked Questions
Q1: Can a model have high accuracy but low precision?
A1: Yes, a model can achieve high accuracy by correctly classifying most instances. However, if it struggles to accurately predict positive cases, its precision could be low, leading to false positives.
Q2: Why is the F1 score preferable for imbalanced datasets?
A2: Imbalanced datasets skew accuracy metrics. The F1 score considers both false positives and false negatives, making it a robust choice for scenarios where one class is dominant.
Q3: How does AUC-ROC help evaluate models?
A3: AUC-ROC offers a comprehensive view of a model’s ability to discriminate between classes at different thresholds. It helps assess overall performance without being affected by class distribution.
Q4: What’s the significance of resource usage in AI models?
A4: Resource-efficient models are cost-effective and environmentally friendly. They enable smoother deployment on edge devices and enhance scalability without compromising performance.
Q5: Is there an ultimate metric for AI performance assessment?
A5: No single metric suits all scenarios. The choice of metrics depends on the application’s requirements, balancing accuracy, efficiency, and specific objectives.